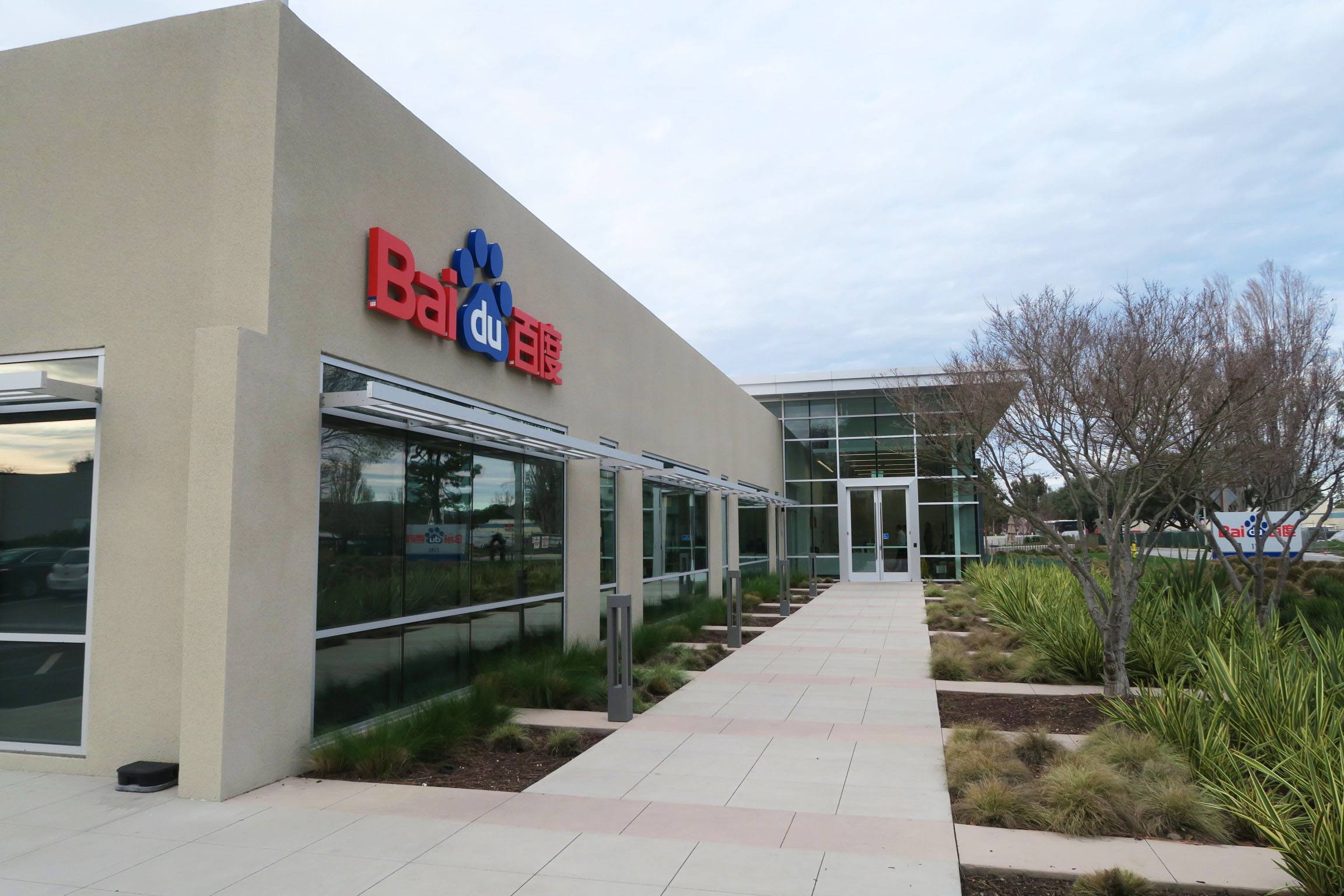
Baidu’s Silicon Valley AI Lab is based in Sunnyvale, California. Photo credit: Tech in Asia.
If it wasn’t for Baidu’s logo, the AI research lab of one China’s largest tech companies would be all but hidden inside a one-story, sand-colored building in Sunnyvale, California. Inside, the lab has the familiar trimmings of a Silicon Valley tech company: a snack bar, standing desks, meeting rooms with grandiose names like “The Great Wall.”
“The reason that the Silicon Valley AI Lab exists is to try to […] find the technology that could get a product to a level where you trust speech recognition the same way you trust a person to understand you,” Adam Coates, director of Baidu’s Silicon Valley AI Lab, tells Tech in Asia. “Our topline goal is to solve speech recognition.”
The AI industry could make or break the future of tech companies around the world.
Baidu wants to build a speech recognition engine that’s 99 percent accurate, a threshold that Andrew Ng, chief scientist at Baidu and founder of Google’s “Google Brain” deep learning project, believes will fundamentally change how humans interact with computers.
“Over the next couple of years, we want to have a software solution that can actually solve that problem,” says Adam.
Estimated to be worth US$16 billion by 2022, the artificial intelligence industry, which includes image recognition and autonomous driving, could make or break the future of tech companies around the world. US tech giants such as Google and Amazon are all heavily invested in their own AI initiatives. Alexa, Amazon’s virtual assistant and Siri competitor, powers Echo, its home assistant gadget; Google’s AI is astonishingly skilled at Pictionary.
See: Half the work people do can be automated: McKinsey
Acquiring AI startups is also a rising trend among large tech firms, which are eager to boost their AI capabilities. In 2014, Google bought DeepMind, the AI startup behind AlphaGo, for more than US$500 million. In January, Microsoft acquired Maluuba, a Canadian startup focusing on natural language processing and general artificial intelligence.
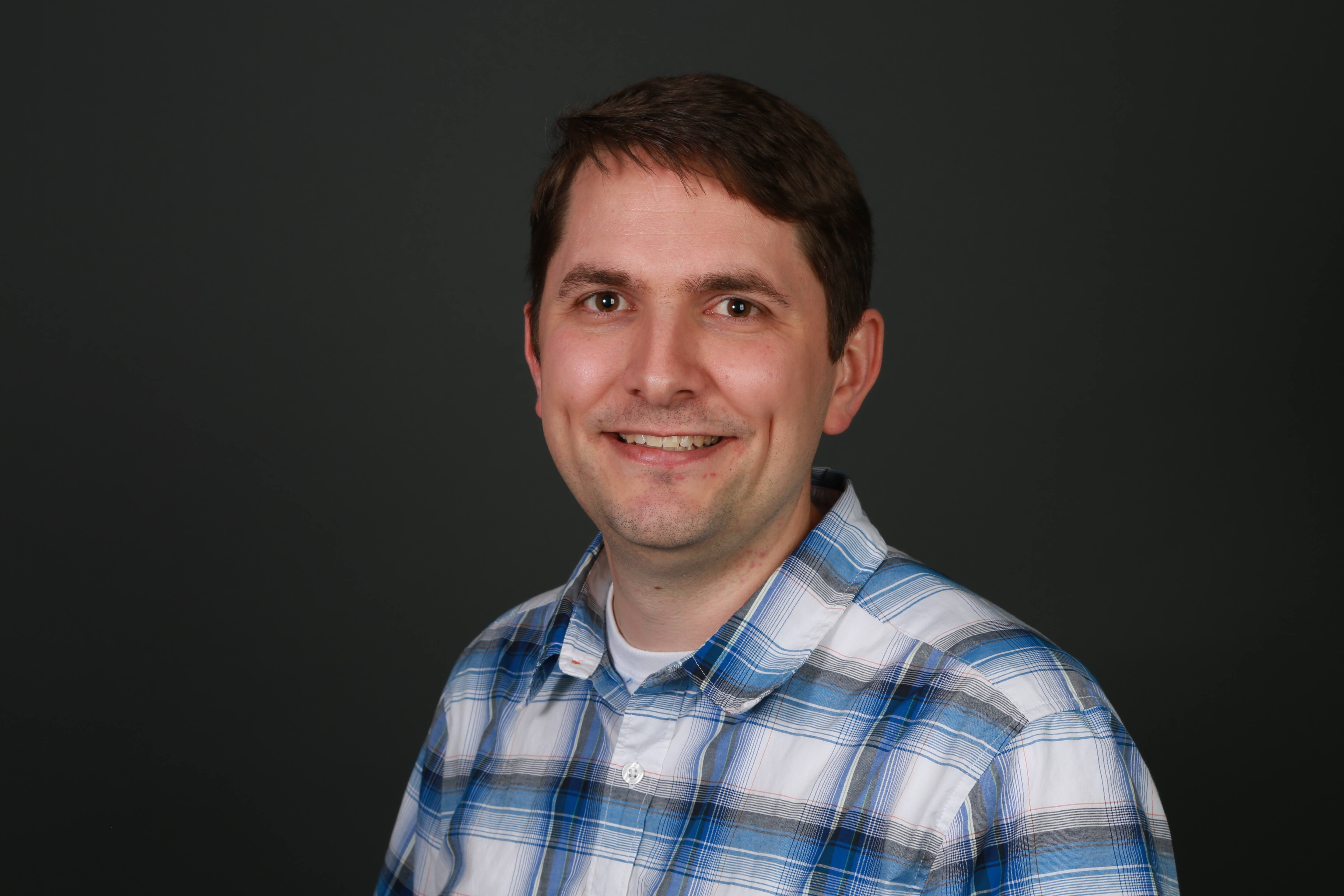
Adam Coates, director of Baidu’s Silicon Valley AI Lab, met Andrew Ng as an undergraduate student at Stanford University. After a machine learning project on teaching helicopters to fly on their own, he was hooked. Photo credit: Baidu.
Baidu, which opened its Silicon Valley AI Lab in 2014, is hoping to carve out a space for itself as a leader in speech recognition. So far, it’s making impressive headway. The company’s latest speech recognition engine, dubbed Deep Speech 2, uses deep learning to recognize words spoken in English and Mandarin, at times outperforming humans in the latter, according to Baidu.
“We can train this giant neural network that eventually learns to recognize speech on its own as well as a human can, and not spend so much of our time thinking about how words are structured,” says Adam. “Instead, [we] can just ask the computer system to learn those things on its own.”
Crunching data
The short answer to Baidu’s plan to conquer speech recognition is data – lots of it. Adam says Deep Speech 2 was trained on tens of thousands of hours of audio recordings. Some of it comes from public data, while another portion is from crowdsourcing services, such as Mechanical Turk, Amazon’s marketplace for odd jobs that require human intelligence.
Baidu’s speech recognition engine was trained on tens of thousands of hours of audio recordings.
“It turns out that even just having people read things to you is very valuable,” Adam explains. It can introduce accents, common mispronunciations, or words with unusual spelling to Baidu’s speech recognition engine, he says.
Deep Speech 2 is an example of supervised learning, a type of machine learning that uses labeled training data – such as transcribed audio – to teach a system new skills, like recognizing handwritten numbers. Without labeled training data, however, the neural network wouldn’t be able to differentiate right from wrong.
“Getting those labels is one of the big expenses and the big challenges of getting stuff like [Deep Speech 2] to work,” says Adam. “It’s not cheap.”
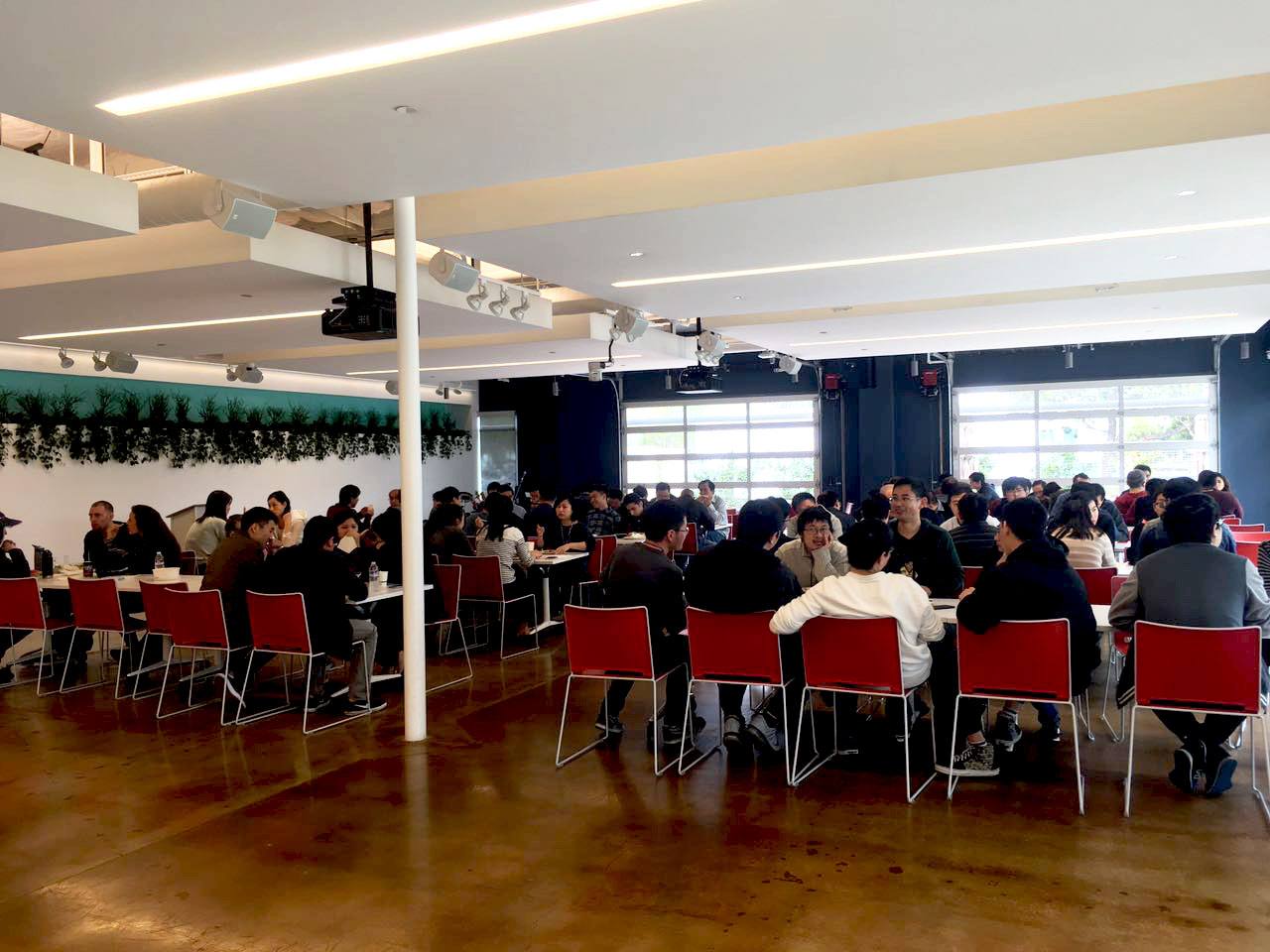
Lunchtime at Baidu’s Silicon Valley AI Lab. The California-based research institute currently has about 50 employees. Photo credit: Tech in Asia.
These labeled audio recordings are fed directly to Deep Speech 2’s neural network in a method known as “end-to-end training.” Unlike more traditional machine learning methods, which break audio data down into discrete units of sound – phonemes – to build the right models, Deep Speech 2’s neural network is language agnostic. It doesn’t need to know anything about the language itself in order to come up with the right algorithm for speech recognition – it just needs a sufficient amount of data.
“As you gave us more data – more and more audio coming in – my machine learning algorithm would get better and better for awhile, and then it would […] just hit a wall,” says Adam, describing an earlier version of Deep Speech, which didn’t use end-to-end training.
Back then, in order to improve accuracy, Baidu had to hire linguists to help tweak and tune its machine learning algorithm. In contrast, Baidu’s latest version of speech recognition uses the same algorithm for both Mandarin and English.
“What’s really amazing about deep learning is that […] if you give a team more data and a bigger computer to crunch it with, deep learning doesn’t seem to hit that same barrier,” he says.
More with less
However, requiring thousands of hours of data to build a deep learning system isn’t realistic for all applications, especially those with a small user base. Finding enough audio data for Thai or a regional dialect in China is significantly more difficult than English, for instance.
“If you launched in a new language, you wouldn’t want to be forced to collect 100,000 hours [of audio] or something crazy,” says Adam. “You’d want to have models that could become very effective with a small amount of data, if possible.”
Deep Speech 2 has other drawbacks that are linked to its dependence on high volumes of data. Even though it becomes increasingly accurate with more data, it can still stumble over words like Tchaikovsky, which are rare yet significant. Adding these outliers to Baidu’s speech recognition engine can require an extraordinary – and costly – amount of data.
“We believe that the amount of data that we might need to handle all of these, say proper names in the world, might just be too uneconomical,” says Adam. Figuring out more efficient speech recognition models will be a top priority for this year and future versions of Deep Speech.
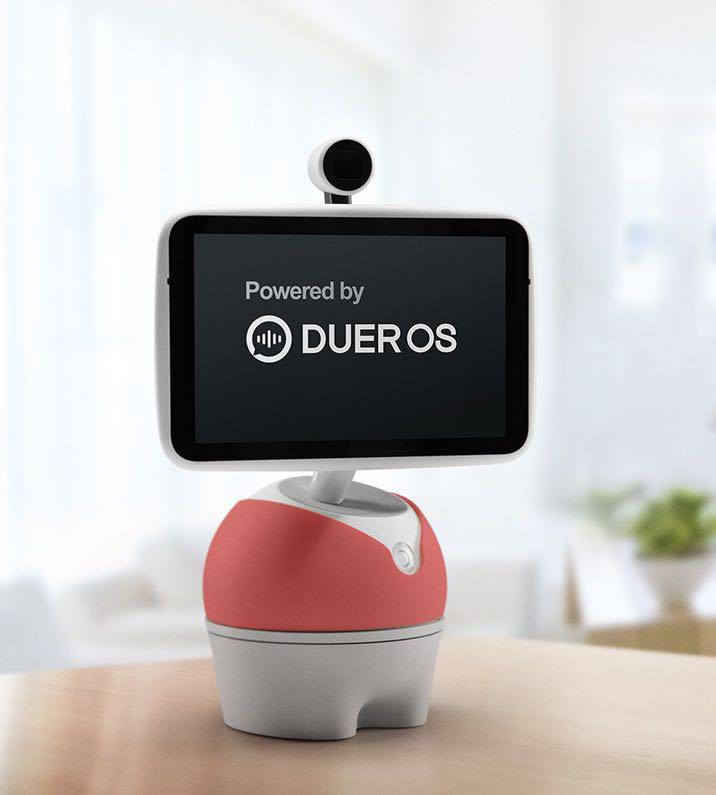
“Little Fish”, the Echo-like home assistant for the Chinese market. Photo credit: Baidu.
Of course, in the end, the research at the Silicon Valley AI Lab ties back to the Baidu’s business. The company’s speech recognition engine is already in several Baidu apps, such as Duer, its Siri-equivalent, as well as Melody, a chatbot that assists doctors with recommendations and treatment options.
Baidu has also developed its own conversational AI platform called DuerOS, which is used by hardware partners to power speech recognition and natural language processing. Chinese hardware company AiNemo, for example, is using DuerOS for its Echo-like home assistant or “Little Fish.”
Still, the Silicon Valley AI Lab is somewhat insulated from Baidu’s commercial side. Across the ocean, just south of Stanford University, Adam and his team can focus their attention on more fundamental research, rather than the kind of work that suffers from tight product deadlines. Baidu’s Beijing-based AI team, on the other hand, is more closely involved with the company’s users and business units, responsible for products like Duer and DuerOS.
“Since we’re further from the products, […] that gives us the freedom to think a little bit about how do we close the gap with humans, which is a much bigger leap,” says Adam.
This post Making ‘Her’ a reality: how Baidu’s AI Lab plans to solve speech recognition appeared first on Tech in Asia.